Digital Twin Tool aims to develop the digital technologies required to operate the tools developed under Modeling the Joint Management of Energy and Water and Socio-Economic and Environmental Aspects. It aims at incorporating, into a holistic way, climate change’s underlying drivers (e.g., energy use, water availability, mobility) from a detailed multi-sectoral data acquisition including critical urban infrastructures, such as energy, water, buildings and mobility, to create a multi-scale virtual or digital twin running on a large-scale IT infrastructure or datacenter. This digital twin will enable the analysis of data and monitoring of urban systems to head off problems before they even occur, prevent downtime, develop new opportunities and planning strategies for the future. Based on an in-depth exploration of co-design of IT infrastructure to execute efficiently advanced numerical models, and using real-time field data, this new digital twin technology can reveal complex patterns of optimization for highly complex urban systems.
This solution will valorise a large-scale computing infrastructure at the EPFL. This complete computing infrastructure at EPFL includes in the DC design 20k servers. The server and storage power consumption is within a total between 10 to 15 kW. This complete modeling infrastructure enables modeling and development of complex management schemes and power behaviors of large-scale computing infrastructures. Also, we include a complete three-layered tree network topology model of a ToR type, aggregation-layer switches. This framework has been used for the development of virtual twins of the digital infrastructure of Credit Suisse including more than 7000 servers in three distributed datacenters in Switzerland.
Tasks included are:
- Design a configurable digital twin framework to support the multi-scale models to scale in computational complexity, and consistent model updates and interoperability across scales.
- Develop a sustainability-oriented methodology for balancing data acquisition with consumed energy on edge AI sensors to update the state of the virtual twin.
- Implement learning approaches for a single or multiple IT infrastructure locations to develop edge-to-cloud solutions for model order reduction and digital twin updates.
- Develop and implement tailored learning techniques that simultaneously preserve performance and privacy.
- Develop approaches that will protect the integrity of edge sensing, and the integrity and confidentiality of raw data and computation in the cloud.
Research Partners
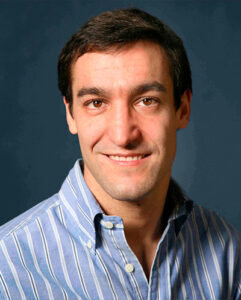
David Atienza
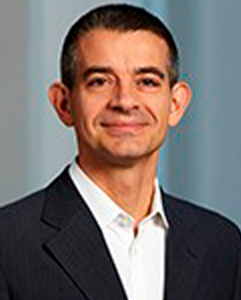
Luca Benini
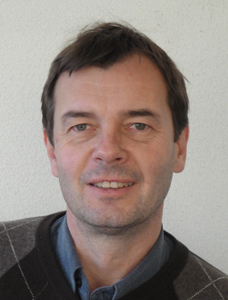
Patrik Hoffmann
Research Laboratory for Advanced Materials Processing
Monitoring of water consumption and quality
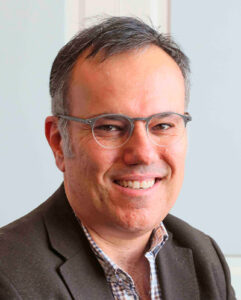
Babak Falsafi
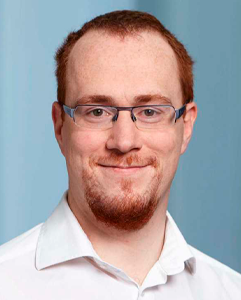
Torsten Hoefler
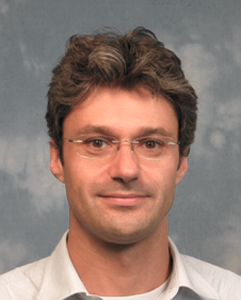
Fabio Nobile
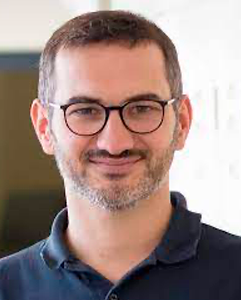
Srdjan Capkun
Publications
Energy-Efficient Frequency Selection Method for Bio-Signal Acquisition in AI/ML Wearables | |||
Taji, Hossein; Miranda Calero, José Angel; Peon Quiros, Miguel; Atienza Alonso, David | |||
2024-07-04 | ISLPED '24: Proceedings of the ACM/IEEE International Symposium on Low Power Electronics and Design | ||
M2SKD: Multi-to-Single Knowledge Distillation of Real-Time Epileptic Seizure Detection for Low-Power Wearable Systems | |||
Baghersalimi, Saleh; Amirshahi, Alireza; Forooghifar, Farnaz; Teijeiro, Tomas; AMIR AMINIFAR; Atienza Alonso, David | |||
2024-05-29 | ACM Transactions on Intelligent Systems and Technology | ||
SAT-based Exact Modulo Scheduling Mapping for Resource-Constrained CGRAs | |||
Tirelli, Cristian; Sapriza, Juan; Rodríguez Álvarez, Rubén; Ferretti, Lorenzo; Denkinger, Benoît Walter; Ansaloni, Giovanni; Miranda Calero, José Angel; Atienza Alonso, David; Pozzi, Laura | |||
2024-04-08 | ACM Journal on Emerging Technologies in Computing Systems | ||
CloudProphet: A Machine Learning-Based Performance Prediction for Public Clouds | |||
Huang, Darong; Costero Valero, Luis Maria; Pahlevan, Ali; Zapater Sancho, Marina; Atienza Alonso, David | |||
2024-01-23 | IEEE Transactions on Sustainable Computing | ||
Enhancing Neural Architecture Search with Multiple Hardware Constraints for Deep Learning Model Deployment on Tiny IoT Devices | |||
A. Burrello, M. Risso, B. A. Motetti, E. Macii, L. Benini, D. J. Pagliari | |||
2023-10-10 | IEEE Transactions on Emerging Topics in Computing | 1-15 | |
Speeding up System Identification Algorithms on a Parallel RISC-V MCU for Fast Near-Sensor Vibration Diagnostic | |||
A. Moallemi, R. Gaspari, F. Zonzini, L. De Marchi, D. Brunelli and L. Benini | |||
2023-09-01 | IEEE Sensors Letters | vol. 7, no. 9, pp. 1-4 | |
Decentralized Federated Learning for Epileptic Seizures Detection in Low-Power Wearable Systems | |||
Baghersalimi, Saleh; Teijeiro, Tomas; Aminifar, Amir; Atienza Alonso, David | |||
2023 | IEEE Transactions on Mobile Computing |